How it Works
At Insectonomy.org, we apply an interdisciplinary approach to explore and manage the roles of insects in sustainable agrifood systems. Our research framework integrates unsupervised machine learning methods to evaluate insect species across different dimensions, focusing on their productive potential, ecosystem potential, use, and the challenges they pose.
Introduction
Insects provide critical socio-ecological roles, and their sustainable use can contribute to solving global challenges in food production, biodiversity conservation, and economic development. However, harnessing their full potential requires careful evaluation of each species' roles in various ecosystems and their ability to meet human needs. Our framework assesses these roles by considering productive, ecological, and social factors while addressing potential challenges like regulatory issues and public acceptance.
Since quantitative information regarding insects' socio-ecological roles is scarce and there are many gaps, a transdisciplinary team of experts in animal sciences, biology, and engineering proposes a qualitative approach to evaluate the productive potential, ecosystem potential, use, and challenges of insect species.
We define:
- Productive Potential (PP) as the insect's ability to be used for productive purposes in agriculture or insect farming.
- Ecosystem Potential (EP) as the insect's contribution to ecological systems and processes.
- Use (U) as the insect's ability to meet human needs and preferences through practical, cultural, and environmental applications.
- Challenges (C) as the obstacles and risks insects pose, including regulatory barriers, pests, toxins, and public acceptance issues.
Methods
Variables Used
To evaluate each species, we categorized them into four key areas, with the following variables for assessing Use, Productive Potential, Ecosystem Potential, and Challenges in the study.
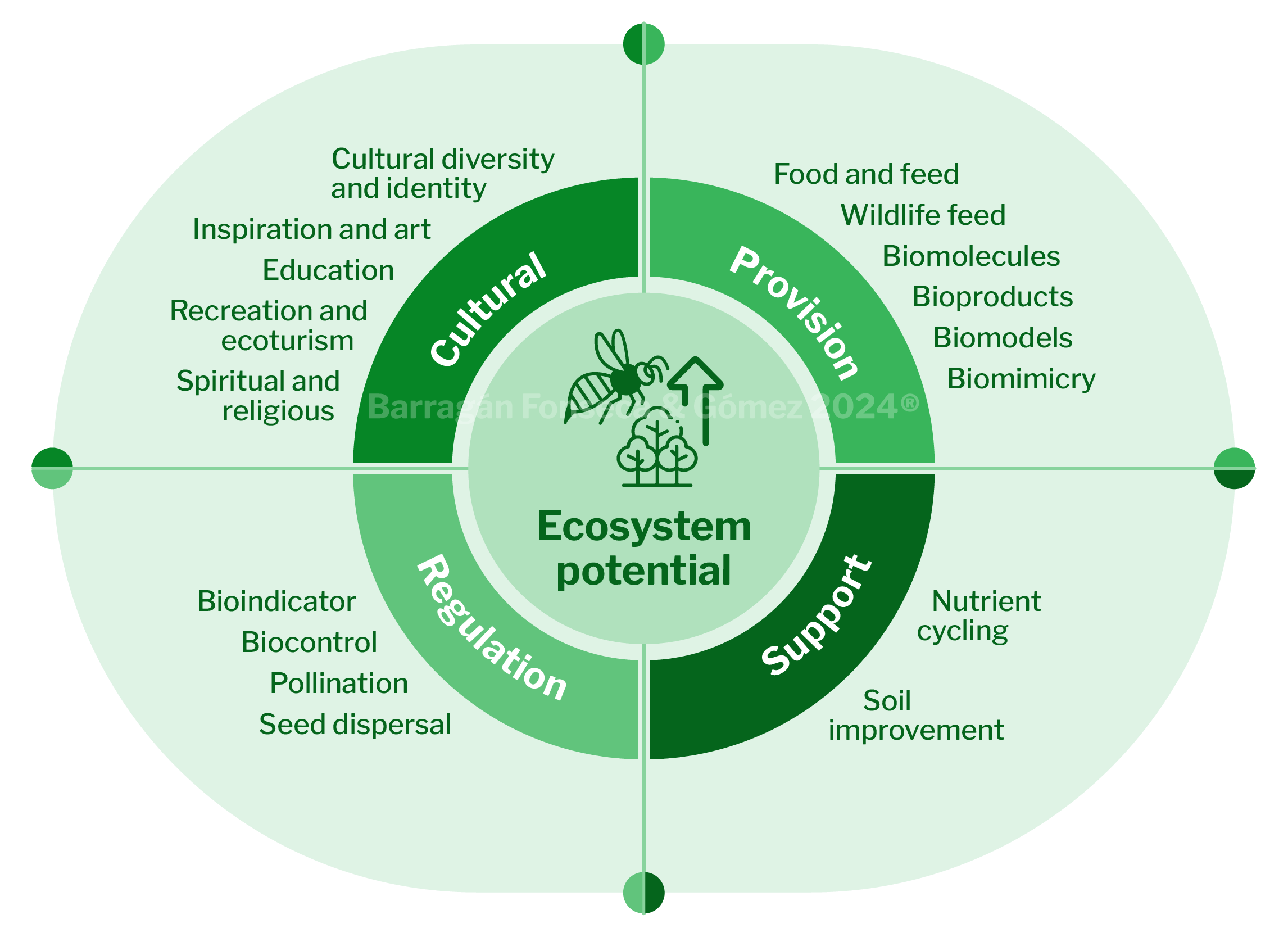
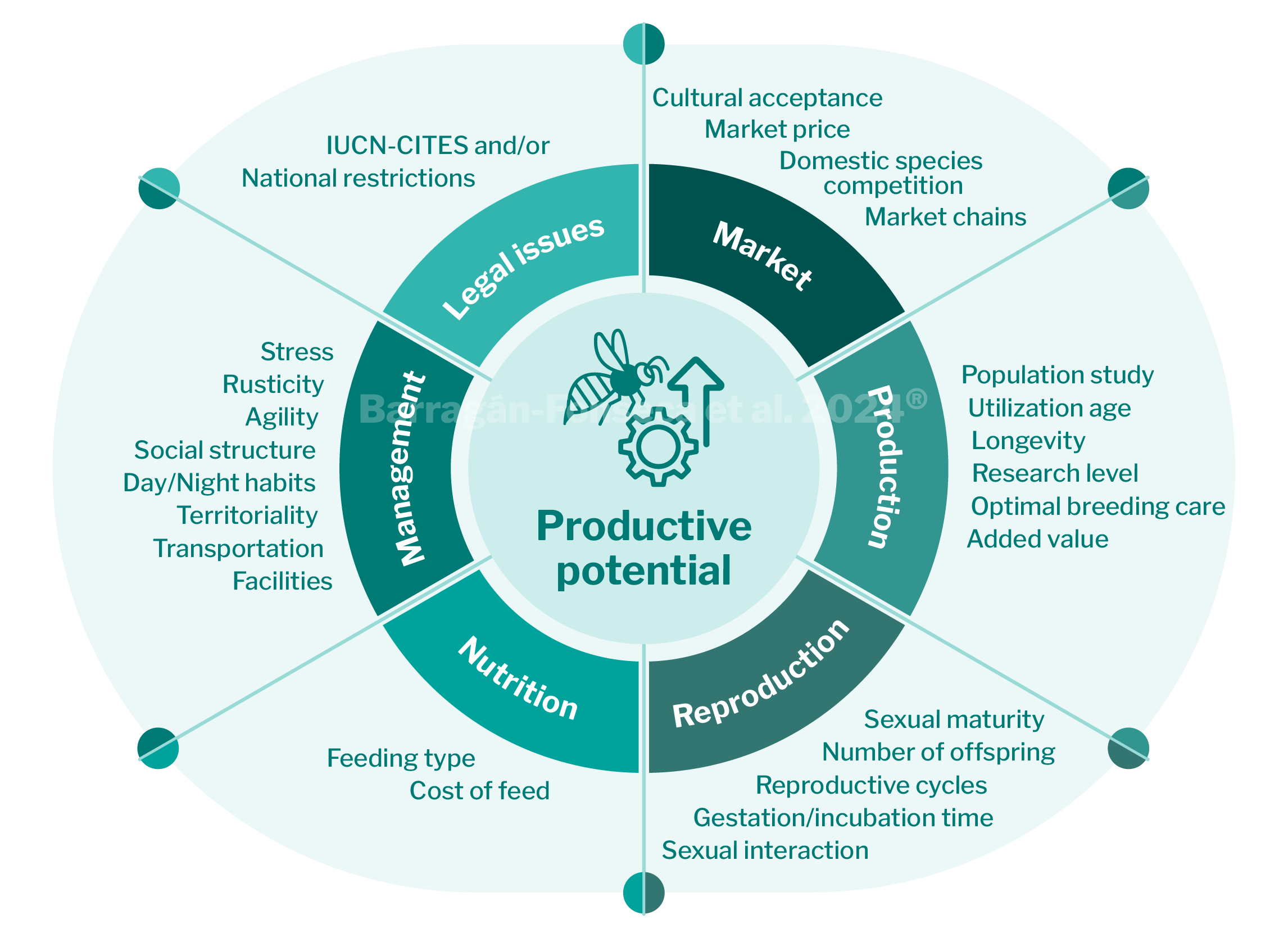
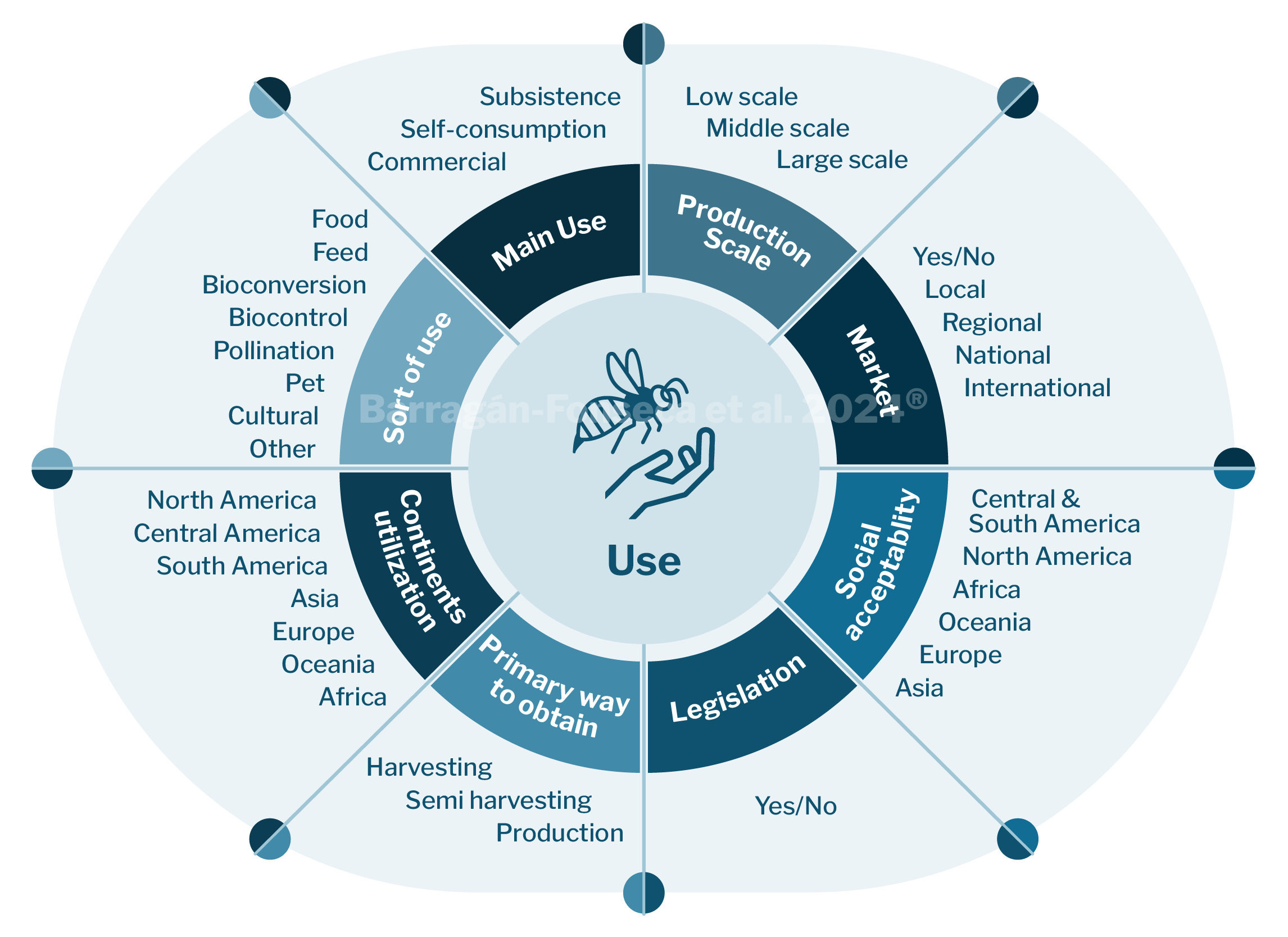
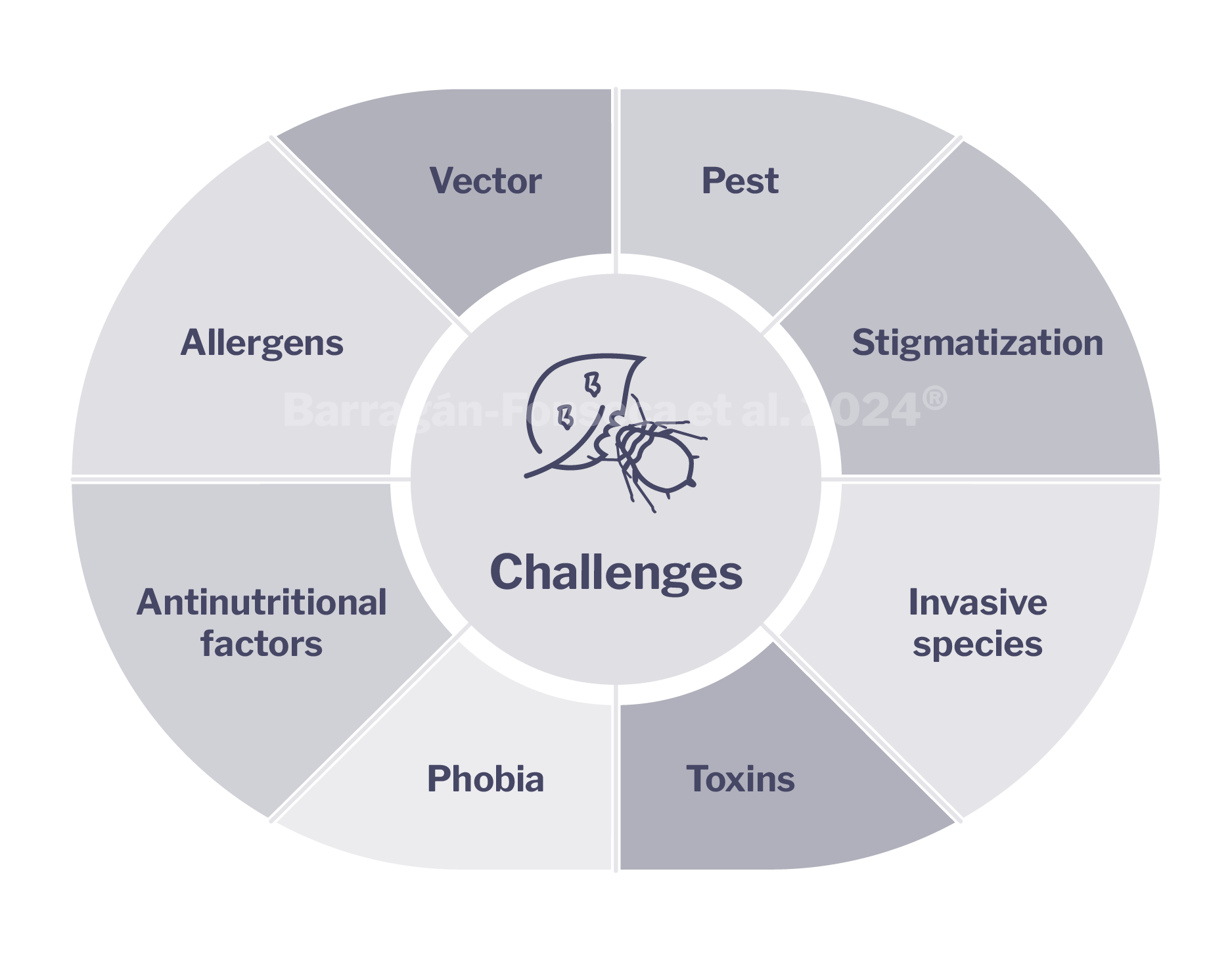
Scoring variables
We qualitatively evaluated categorical variables by assigning scores to selected variables, allowing each to reflect a favourable or unfavourable value for specific characteristics. Each category includes variables rated based on productive or ecosystem favourability, the use of the species, and challenges. Each variable is scored from 0 to 3, where 0 is the least favourable and 3 is the most favourable, as proposed by Barragán-Fonseca (2011) and Barragán-Fonseca and Hermann-Rodríguez (2019) for evaluating wild animals. An exception is made for challenges, where higher scores indicate less favourability. Scores were estimated qualitatively through a consensus-based questionnaire administered to the research team, relying on causal relationships, expertise, and literature reviews. Additionally, we recorded the geographical, biological, and ecological characteristics of each insect species.
Selection index
We created selection indices using the PROMETHEE method (Preference Ranking Organization Method for Enrichment Evaluation), a multi-criteria decision-making tool. This method combines various criteria to rank and evaluate alternatives, providing scores from low to high potential across 120 insect species. We rescaled these indices to a 0-100 scale for easy interpretation. Each species receives a score from 0-100 in the categories of Use, Production Potential, Ecosystem Potential, and Challenges. Higher scores indicate greater potential in the first three categories, while in Challenges, a higher score reflects greater difficulty in utilization.
Unsupervised Machine Learning Analysis
Data from the 120 species were organized into a numerical matrix, with rows as species and columns as variables, enabling calculations of relative distances using Euclidean metrics. Clustering techniques grouped species based on similarities across PP, EP, Use, and Challenges.
We used UMAP (Uniform Manifold Approximation and Projection) to reduce 115 variables to two dimensions, preserving local and global structures for better visualization. DBSCAN (Density-Based Spatial Clustering) identified dense clusters and flagged outliers. This unsupervised analysis revealed intra-class similarities and inter-class differences, providing an objective, data-driven framework to classify species for insect management.
Insights & Outlook
Unsupervised machine learning grouped insect species by productive potential, ecosystem services, usage patterns, and challenges, revealing roles in agrifood systems and barriers like cultural or regulatory issues. Findings highlight insects' potential in food, ecosystem support, and niche markets, requiring tailored approaches to address regional and cultural factors.
Future steps include expanding datasets, refining algorithms, and incorporating broader socio-ecological variables. Collaboration will enhance strategies for sustainable insect use, integrating promising species into agrifood systems while managing risks.
To explore the data and access visual insights, visit the "Species Data Explorer" tab on the website. Extended details are included in the submitted manuscript: "Barragán-Fonseca, K.B., Ortiz, J.E., Giron, D., & García-Arteaga, J.D. (submitted). Evaluating insect species for sustainable agrifood systems: An integrated clustering analysis", which will soon be published on the webpage.